Keynote Speakers
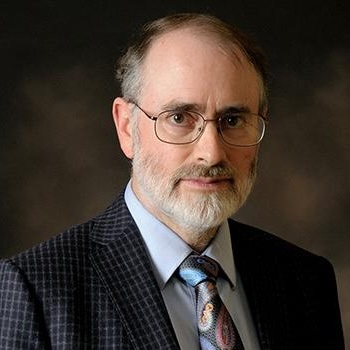
Prof. Thomas G. Dietterich
Oregon State University, USA
Prof. Dietterich (AB Oberlin College 1977; MS University of Illinois 1979; PhD Stanford University 1984) is Distinguished Professor Emeritus in the School of Electrical Engineering and Computer Science at Oregon State University. Dietterich is one of the pioneers of the field of Machine Learning and has authored more than 220 refereed publications and two books. He is the recipient of the 2024 IJCAI Award for Research Excellence. His current research topics include robust artificial intelligence, robust human-AI systems, and applications in sustainability. Dietterich has devoted many years of service to the research community and was recently given the ACML Distinguished Contribution and the AAAI Distinguished Service awards. He is a former President of the Association for the Advancement of Artificial Intelligence and the founding president of the International Machine Learning Society. Other major roles include Executive Editor of the journal Machine Learning, co-founder of the Journal for Machine Learning Research, and program chair of AAAI 1990 and NIPS 2000. He currently oversees the Computer Science categories at arXiv.
Integrating machine learning into safety-critical systems
The impressive new capabilities of systems created using deep learning are encouraging engineers to apply these techniques in safety-critical applications such as medicine, aeronautics, and self-driving cars. This talk will discuss the ways that machine learning methodologies are changing to operate in safety-critical systems. These changes include (a) building high-fidelity simulators for the domain, (b) adversarial collection of training data to ensure coverage of the so-called Operational Design Domain (ODD) and, specifically, the hazardous regions within the ODD, (c) methods for verifying that the fitted models generalize well, and (d) methods for estimating the probability of harms in normal operation. There are many research challenges to achieving these. But we must do more, because traditional safety engineering only addresses the known hazards. We must design our systems to detect novel hazards as well. We adopt Leveson’s view of safety as an ongoing hierarchical control problem in which controls are put in place to stabilize the system against disturbances. Disturbances include novel hazards but also management changes such as budget cuts, staff turnover, novel regulations, and so on. Traditionally, it has been the human operators and managers who have provided these stabilizing controls. Are there ways that AI methods, such as novelty detection, near-miss detection, diagnosis and repair, can be applied to help the human organization manage these disturbances and maintain system safety?

Prof. Emanuele RodolÃ
Sapienza University of Rome, Italy
Prof. Emanuele Rodolà is a Full Professor of Computer Science at Sapienza University of Rome, where he leads the GLADIA group focusing on Geometry, Learning, Audio, and Applied AI. His work in this area is funded by an ERC Starting Grant and a Google Research Award. Before his current position, he served as an Assistant and then Associate Professor at Sapienza (2017-2020), a postdoc at USI Lugano (2016-2017), an Alexander von Humboldt Fellow at TU Munich (2013-2016), and a JSPS Research Fellow at The University of Tokyo (2013), in addition to visiting periods at Tel Aviv University, Technion, Ecole Polytechnique, and Stanford. He is a fellow of ELLIS and the Young Academy of Europe. Prof. Rodolà has received several awards for his research, he has been active in the academic community, serving on program committees and as an area chair for leading conferences in computer vision, machine learning, and graphics (CVPR, ICCV, ICLR, NeurIPS, etc.). His current research mainly focuses on neural model merging, representation learning, ML for audio, and deep multimodal learning, and has published around 150 papers in these areas.
Unlocking Neural Composition
As human beings, we spend a significant portion of our lives learning from our predecessors; it is a fruitful, but ultimately inefficient process. What if we could share knowledge instantaneously, at the same time bypassing the barriers of language? This breakthrough would accelerate scientific progress, avoid redundant rediscoveries while preventing miscomprehension, ultimately transforming science into a truly collective effort. Alas, human brains are not amenable to such forms of communication -- but deep learning models can. In this talk I'll report an important (and somewhat surprising) empirical observation: under the same data and modeling choices, distinct latent spaces typically differ by an unknown quasi-isometric transformation; that is, in each space, the distances between the encodings do not change. I'll then show how simply adopting pairwise similarities as an alternative data representation leads to guaranteed isometry invariance of the latent spaces, effectively enabling instantaneous latent space communication: from zero-shot model stitching to latent space comparison between diverse settings. Several validation experiments will follow on different datasets, spanning various modalities (images, text, graphs), tasks (e.g., classification, reconstruction) and architectures (e.g., CNNs, GCNs, transformers).